AI Is Bluffing (And You’re Buying It)
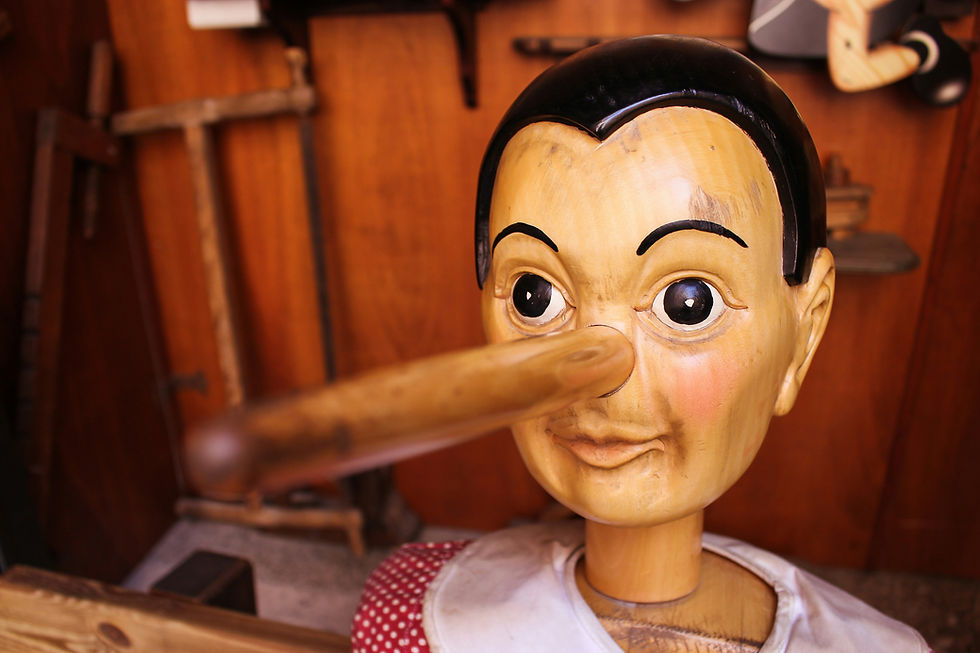
It Sounds Smart, But It’s Guessing: How Generative AI Really Works
In a world increasingly enchanted by artificial intelligence, it’s easy to forget one important truth: Generative AI doesn’t understand what it’s saying.
Whether it’s writing a speech, answering a legal question, or drafting a business strategy, tools like ChatGPT, Gemini, or Claude don’t know what they're doing. They’re not intelligent in the way we are. They’re sophisticated pattern matchers—algorithms trained to predict the next word based on mountains of data.
It’s impressive, yes. But it’s also misleading. And sometimes, dangerous.
So… How Does It Really Work?
Generative AI is powered by large language models (LLMs). These models are trained on vast collections of text—books, articles, code, social media, and more. The goal? To learn how humans typically use language, and then replicate that with uncanny fluency.
When you type a prompt like “Summarize the French Revolution,” the AI doesn’t go digging through history books. Instead, it calculates:
“Based on everything I’ve seen during training, what’s the most statistically likely next word, phrase, or paragraph to follow this prompt?”
That’s it. No understanding of France. No sense of history. No grasp of political upheaval.
It’s more like a hyper-sophisticated autocomplete engine than a virtual historian.
The Big Flaw: Confidently Wrong
Because Gen AI is built to sound good, not be right, it frequently produces content that’s grammatically perfect—but factually wrong.
And here’s the kicker: it delivers these mistakes with total confidence.
Let’s look at some real examples:
🔹 Case 1: The Phantom Research Paper
A researcher asked an AI to list recent studies on a rare disease. It gave five convincing citations. When the researcher looked them up—none existed. The paper titles were fake. The authors were real academics, but they hadn’t written those works. A dangerous fabrication, especially in fields like medicine.
🔹 Case 2: The Wrong Formula
An AI-generated Excel solution for a client’s marketing funnel included a statistical formula for calculating conversion lift. It looked plausible—but the math was flawed, leading to a 12% overestimation of ROI.
🔹 Case 3: The Made-Up Law
A paralegal used an AI tool to draft a legal brief. It cited a case that had never been tried. The structure of the case law looked real, even the citation format was correct. But when submitted, the court flagged it as entirely fabricated.
These aren’t just one-off failures. They stem from how these systems are built: to predict the most likely answer, not the most correct one.
Why This Matters More Than Ever
In low-risk situations—like generating jokes, writing poetry, or naming your fantasy football team—a wrong answer is just a minor blip.
But in real-world decision-making, the consequences can be severe:
In Business: Poor financial decisions based on incorrect forecasting or analysis.
In Education: Students learning false information and citing hallucinated sources.
In Healthcare: Dangerous treatment suggestions based on unreliable summaries.
In Law: Legal professionals presenting fabricated precedent or flawed arguments.
In HR or Recruitment: Biased or misleading summaries of candidates or job descriptions.
And because Gen AI sounds so fluent and polished, it gives people a false sense of authority. If it sounds intelligent, it must be intelligent… right? Wrong. This illusion of understanding is perhaps the most dangerous part of all.
Four Practical Safeguards
Generative AI isn’t going away—and we shouldn’t want it to. It’s a powerful tool that can boost productivity, creativity, and learning. But we need to use it with open eyes and sharp minds.
Here are four things individuals and organizations should put in place:
✅ 1. Always Fact-Check
Don’t trust, verify. Use AI as a springboard, not a source of truth. If a number, name, or quote appears—double-check it from a credible source.
✅ 2. Prompt Smarter, Think Sharper
The better your prompt, the better your output—but no prompt will guarantee accuracy. Critical thinking must always follow.
✅ 3. Educate AI Users
We need a new kind of literacy: AI literacy. Users must understand how Gen AI works—its strengths, its blind spots, and when to be skeptical.
✅ 4. Build Human-in-the-Loop Systems
In any professional setting, AI-generated output should go through human review. This isn’t a nice-to-have—it’s essential. Whether you’re generating marketing copy or analyzing data, someone needs to sanity-check the results.
Bonus tip: If the output feels too perfect, that’s your red flag.
Final Thoughts
Generative AI is a powerful co-pilot. It helps us write, think, analyze, and explore faster than ever. But it is not a thinking machine. It does not know right from wrong, true from false, or helpful from harmful.
It mimics intelligence without possessing it.
So let’s embrace the technology—but pair it with human judgment, strong ethics, and a good dose of skepticism. Because the best decisions will always come from a mind that not only reads the answer—but understands the cost of getting it wrong.
Comentarios