From Data Insights to Decisive Action: Bridging the Analytics Value Chain
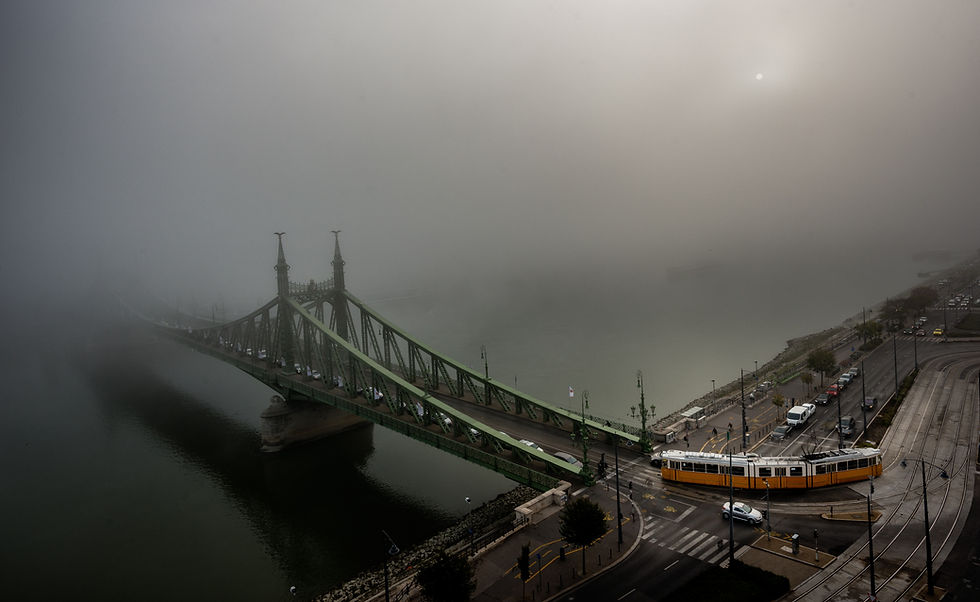
In a world increasingly driven by data, the ability to extract meaningful insights is no longer a differentiator—it’s an expectation. However, transforming those data insights into actionable decisions remains a complex challenge. While data analytics excels at uncovering patterns and validating hypotheses, its true value lies in bridging the gap between insights and action. Most analytics classes emphasize data-related skills but often overlook the critical step of transforming data insights into actionable options. This process requires venturing beyond analytics into the realm of decision sciences, where human judgment, contextual understanding, and structured decision-making come together to bridge the gap between insights and impactful decisions.
Section 1: Turning Data Insights into Actionable Options
Data analytics often highlights correlations and trends—such as declining fertility rates in wealthier countries or leading indicators of heart disease—but it stops short of prescribing actionable solutions. The process of generating actionable options requires more than statistical expertise; it demands contextual knowledge, systems thinking, and an understanding of organizational dynamics. For instance, while analytics might identify key factors contributing to heart disease, crafting a national health policy involves addressing multiple layers of complexity. This includes funding for preventive care, tackling socioeconomic disparities in healthcare access, and managing resistance from industries impacted by potential regulations. These challenges highlight the importance of interdisciplinary collaboration and stakeholder engagement in transforming insights into effective actions.

1. Contextual and Domain Knowledge: The Foundation of Actionable Insights
Turning data insights into options starts with a deep understanding of the domain in which the decision will be made. Contextual knowledge ensures that insights are interpreted correctly and that recommendations are practical, relevant, and feasible.
Understanding Context: Insights are only valuable when viewed through the lens of industry-specific challenges and opportunities. For example, in the energy sector, understanding seasonal demand fluctuations ensures resource allocation strategies are both cost-efficient and reliable. Similarly, in retail, identifying regional buying patterns allows organizations to optimize inventory and meet customer needs effectively. Addressing declining fertility rates highlights the necessity of contextual factors such as cultural norms, economic incentives, and policy interventions, showcasing the need for tailored solutions.
Navigating Constraints: Translating insights into action requires addressing key constraints such as regulatory compliance, operational feasibility, and organizational readiness. These factors ensure recommendations can be effectively implemented within real-world limits.
Applying Domain Expertise: Leveraging specialized knowledge of industry practices and benchmarks enables solutions to align closely with both organizational objectives and broader market dynamics, increasing the likelihood of success.
2. Systems Thinking: Seeing the Bigger Picture
Complex problems rarely exist in isolation. Systems thinking enables analysts to understand interdependencies and anticipate the broader implications of potential actions.
Recognizing Interconnectedness: Many issues involve multiple factors that influence and reinforce each other. For example, a policy addressing heart disease might impact healthcare infrastructure, societal behaviors, and economic resources.
Anticipating Consequences: Effective options require foresight to mitigate unintended outcomes while maximizing intended benefits.
Balancing Short- and Long-Term Goals: Analysts must evaluate immediate solutions against their long-term sustainability and alignment with strategic objectives.
By combining contextual knowledge and systems thinking, analysts can craft actionable options that are both grounded in reality and strategically sound.
Section 2: Facilitating Decision-Making with Decision Science
Once actionable options are developed, presenting them in a way that facilitates decision-making is crucial. Decision sciences provide structured methodologies to evaluate options, clarify trade-offs, and prioritize actions. By blending analytical rigor with stakeholder perspectives, decision sciences transform data insights into clear, actionable choices that foster alignment, build consensus, and empower leaders to act with confidence.
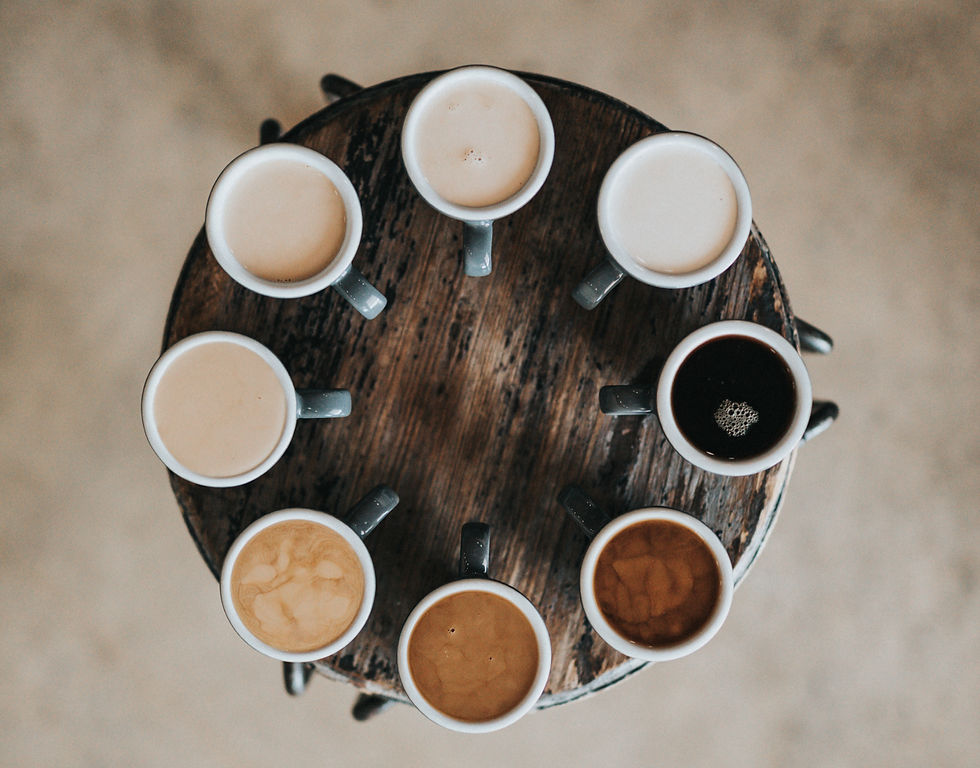
The Goldilocks Principle: Managing the Number of Options
One common challenge is presenting the right number of options. While too few choices can limit creativity, too many can overwhelm decision-makers and lead to analysis paralysis.
Optimal Range: For complex decisions, research shows that 3-5 well-considered options strike a balance between diversity and manageability. Cognitive psychology studies indicate that when decision-makers face too many options, cognitive overload can set in, leading to analysis paralysis or poor decisions. By narrowing the field to a manageable range, analysts ensure that stakeholders can evaluate choices comprehensively without feeling overwhelmed.
Filtering and Prioritization: Analysts should systematically curate options by relevance, impact, and feasibility. Using tools like weighted decision matrices or scoring frameworks helps highlight the most actionable paths. Additionally, prioritization should consider organizational goals and constraints to ensure the final options align with strategic priorities and resource availability, enhancing the likelihood of successful implementation.
Building Consensus Through Effective Framing
Data-driven decisions often require buy-in from diverse stakeholders. Presenting options in a way that aligns with organizational goals and values is key to achieving consensus.
Scenario Planning: Presenting best-case, worst-case, and most-likely scenarios helps stakeholders weigh trade-offs and visualize potential outcomes. For instance, in budget planning, scenario planning can illustrate how different allocation strategies might impact financial stability under varying economic conditions, fostering a deeper understanding of trade-offs.
Decision Matrices: Structured frameworks, such as weighted decision matrices, allow stakeholders to compare options systematically against organizational priorities. These tools can quantify subjective preferences, highlight the strengths and weaknesses of each option, and provide a clear rationale for selecting the most viable choice.
Bias Mitigation: Analysts must proactively address cognitive biases that can distort decision-making. For example:
Action Bias: This bias often drives stakeholders to prioritize action for the sake of appearing decisive, even when the best option is to wait or gather more data. Highlighting the potential risks and costs of premature action can counter this bias.
Status Quo Bias: Stakeholders may resist change due to comfort with the current state or fear of the unknown. Providing evidence-based projections and emphasizing the benefits of change can help overcome inertia and build confidence in new directions.

Evolving Decision-Making Processes: Lessons Learned
Effective decision-making is not static; it evolves with experience and reflection. Organizations should evaluate their decision-making processes to ensure they consistently deliver value over time. This evaluation should focus on the process rather than the outcomes, as outcomes can sometimes result from luck or external factors unrelated to the decision process. For example, a global healthcare organization recently implemented a decision-review framework that incorporated feedback loops. By analyzing past decisions on resource allocation for pandemic preparedness, they identified inefficiencies in their escalation protocols and refined their processes. This iterative approach not only improved response times but also enhanced trust across teams, illustrating the importance of learning from past experiences to refine and evolve decision-making processes.
Focus on Process, Not Perfection: Decision-making should aim for reliable outcomes rather than flawless ones. Any decision is based on the best available information within given constraints.
Learning from Past Decisions: Reviewing past decisions should focus on evaluating the decision-making process itself rather than solely on outcomes. For example, was the process well-structured? Were the right data and tools used? Did stakeholders have clear roles? This distinction is crucial since positive outcomes can sometimes result from luck, while poor outcomes may stem from external factors. By understanding and refining the decision process, organizations can improve consistency and reliability over time.
Avoiding Over-Complexity: Decision frameworks should balance thoroughness with efficiency, especially for time-sensitive issues. Streamlining processes by reducing unnecessary steps or redundancies ensures that decisions are made swiftly and effectively. For example, rapid response protocols used during crises, such as pandemic management, emphasize clarity and speed, enabling organizations to seize opportunities and mitigate risks in real-time.
At FYT Consulting, we specialize in bridging the gap between analytics and decision-making. By integrating contextual knowledge, systems thinking, and decision sciences, we help organizations transform data-driven insights into impactful decisions. Our approach empowers leaders to navigate complexities, build consensus, and drive sustainable outcomes.
The journey from insight to action is both an art and a science. It requires the analytical rigor to uncover patterns, the contextual expertise to craft actionable options, and the decision-making acumen to guide organizations toward their goals. By mastering these elements, organizations can unlock the full value of their data and chart a path to sustained success.
Comments