Predicting the Future: Real-World Applications of Regression Analysis
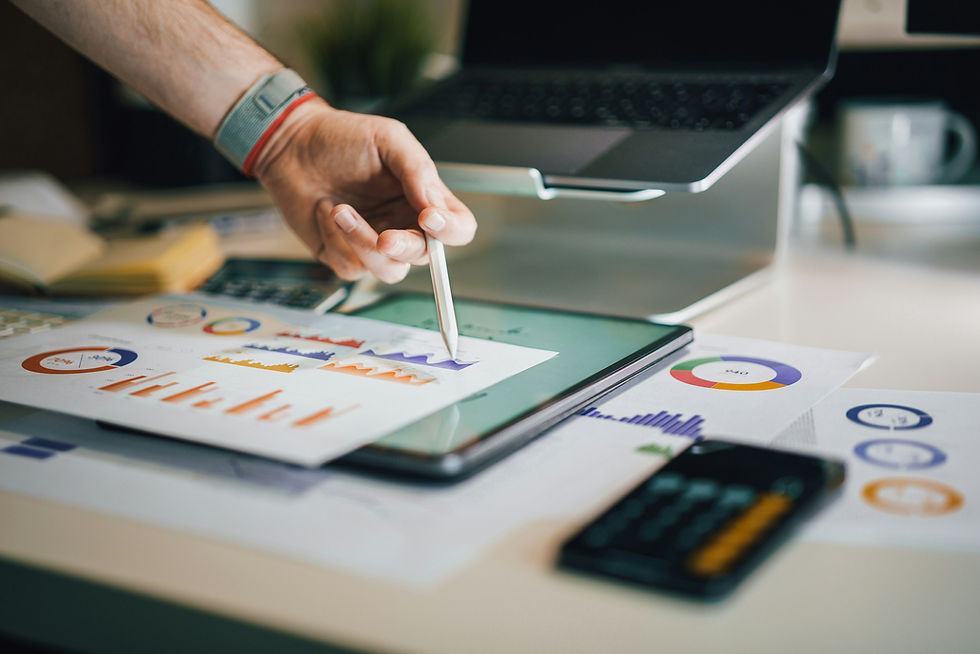
Photo by Jakub Żerdzicki on Unsplash
In the world of data analytics, regression is a powerful and commonly used statistical method. It helps us understand the relationships between variables and make predictions based on historical data. Whether it's predicting future sales, assessing the impact of marketing efforts, or estimating real estate prices, regression analysis provides valuable insights that guide decision-making. This article will explore what regression is, the different types of regression, and three everyday applications where it plays a crucial role.
What is Regression?
Regression analysis is a statistical technique used to examine the relationship between a dependent variable (the outcome) and one or more independent variables (predictors). The goal is to model the relationship so that the dependent variable can be predicted based on the values of the independent variables. Essentially, regression helps us understand how changes in one variable are associated with changes in another.
Types of Regression
1. Linear Regression
Simple Linear Regression: This involves a single independent variable and aims to find a linear relationship between it and the dependent variable. For example, predicting a student's exam score based on the number of hours studied.
Multiple Linear Regression: This involves multiple independent variables to predict the dependent variable. For instance, predicting house prices based on square footage, location, and the number of bedrooms.
2. Logistic Regression
Unlike linear regression, logistic regression is used when the dependent variable is binary (e.g., yes/no, success/failure). It predicts the probability of a certain event occurring. For example, determining whether an email is spam or not based on various features of the email.
3. Polynomial Regression
This type of regression is used when the relationship between the independent and dependent variables is non-linear. Polynomial regression fits a polynomial equation to the data. For instance, modeling the growth rate of bacteria over time, which might follow a non-linear pattern.
Everyday Applications of Regression
Predicting Sales Performance
Application: Retail companies often use regression analysis to predict future sales based on historical sales data, marketing spend, seasonal trends, and economic indicators.
Example: A company might use multiple linear regression to forecast next quarter's sales by analyzing past sales data, advertising expenditures, and economic conditions.
2. Real Estate Price Estimation
Application: Real estate agents and analysts use regression to estimate property values based on various factors like location, square footage, number of bedrooms, and local amenities.
Example: By applying multiple linear regression, an agent can predict the selling price of a house considering its size, neighborhood, proximity to schools, and other relevant features.
3. Healthcare Outcomes
Application: In healthcare, regression analysis helps predict patient outcomes based on medical history, treatment plans, and demographic information.
Example: Logistic regression can be used to predict the likelihood of a patient developing a particular condition based on their age, weight, blood pressure, and family medical history.
Conclusion
Regression analysis is a versatile tool that plays a vital role in various fields by providing insights and predictions based on data. Understanding the different types of regression and their applications can help you leverage this technique effectively in everyday scenarios. Whether it's predicting sales, estimating property prices, or improving healthcare outcomes, regression analysis offers a robust framework for making informed decisions.
Comments